製薬業界における AI: リスク、リターン、そして今後の展望
人工知能(AI)の利用は今やあらゆる業界に浸透しており、製薬業界も例外ではありません。既存のデータに基づいて予測を行う機械学習(ML)モデルや、トレーニングデータに基づいて新しいデータを生成する生成AI(GenAI)モデルなど、AIは研究から承認、マーケティングに至るまで、薬品開発プロセスの各ステップを効率化し、加速するために活用されています。
当社独自のホワイトペーパーで、製薬業界におけるAIについて詳細をご確認いただけます。
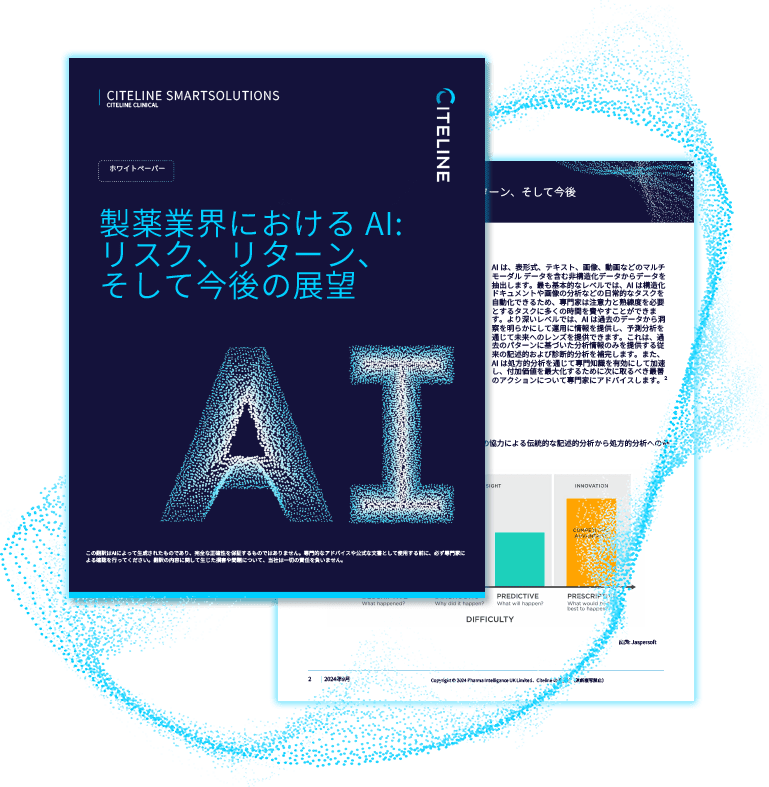