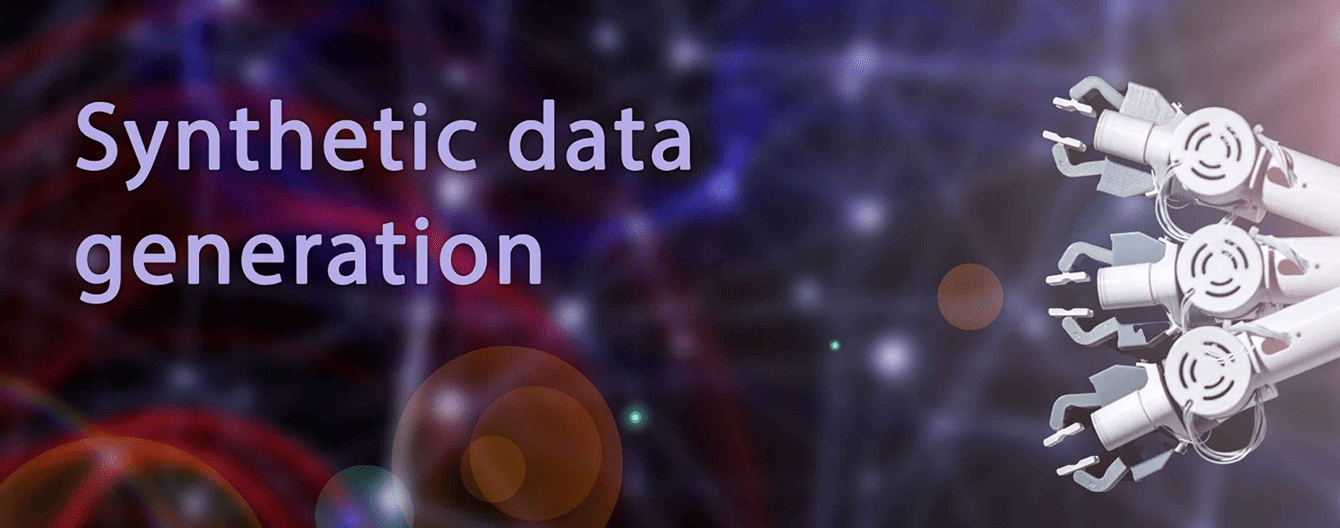
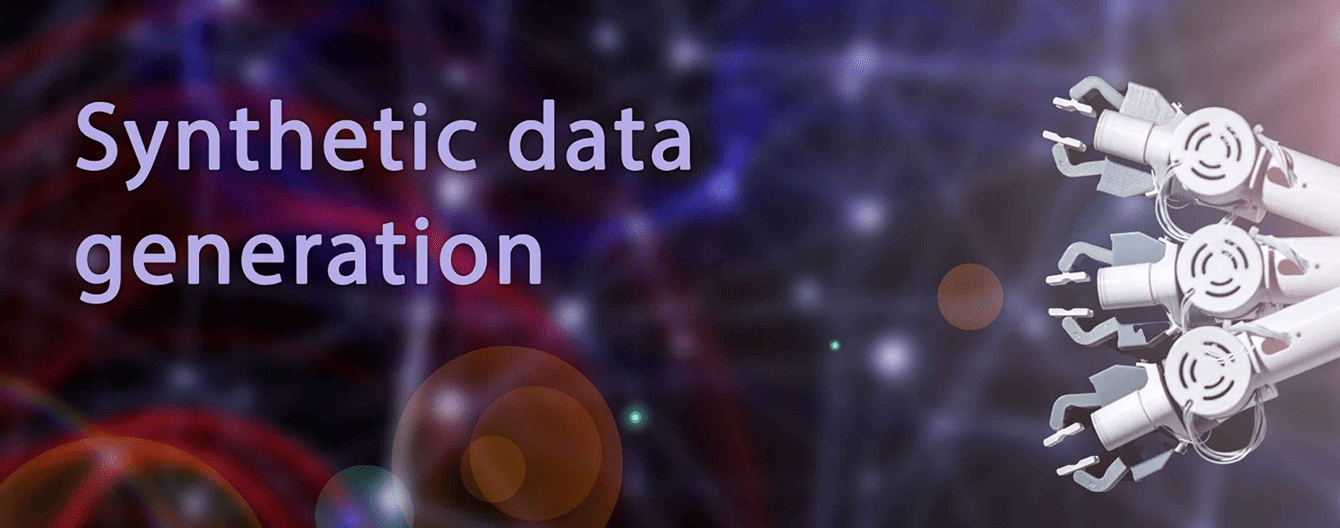
Synthetic data has many applications in clinical research. In this article, we’ll dive into three use cases:
- Protecting patient privacy
- Training AI models
- Filling gaps in RWD
In the pharmaceutical industry today, there’s a lot of buzz around real-world data (RWD). What’s flying under the radar, despite its value in clinical research, is synthetic data.
Life sciences isn’t the only industry benefiting from the use of synthetic data. In the banking industry, artificial data is bolstering artificial intelligence (AI) models used to detect fraud. Because synthetic data can simulate a wide range of scenarios, it can improve testing and validation in manufacturing. The retail industry has applied the use of synthetic data to training and automation. In fact, Gartner estimates that by 2030, the majority of the data used to build AI models will be synthetic data.
Not all AI is created alike. Synthetic data is a form of generative AI. In contrast with traditional AI, which focuses on analyzing and interpreting data, generative AI focuses on creating new content. Diving a little deeper, one type of generative AI is generative adversarial networks (GANs), which uses two competing AI models to produce synthetic data.1
Synthetic data should not be confused with synthetic controls, cohorts of patients from external data and adjusted by employing statistical methodologies, used in clinical trials. In lieu of recruiting patients assigned to a control arm, a synthetic control arm repurposes historical clinical data or RWD to accurately match those patients.
In clinical research, synthetic data also can be used to enhance datasets and increase diversity. Where datasets are imbalanced and not representative of the population they aim to serve, generative AI in the form of synthetic minority oversampling technique (SMOTE) may be used to selectively augment the representation of minority datapoints.1
Another advantage of synthetic data is in relation to safeguarding privacy. A Gartner article expands on this, quoting Alys Woodward, senior director analyst: “Synthetic data can bridge information silos by acting as a substitute for real data and not revealing sensitive information, such as personal details and intellectual property.”
“Synthetic data is a relatively new kind of space, in the past five to seven years or so,” says Brad Davis, a principal in consulting and analytics at Norstella, Citeline’s parent company. “It is a relatively new concept, but one that’s incredibly powerful.” He cites a trio of use cases for clinical research:
Use case #1: protecting patient privacy
Because synthetic datasets have all the same statistical characteristics as the real-world dataset, the same analyses and calculations can be applied. However, synthetic data can randomly generate patient-identifying information which can further protect against patient reidentification. For example, simulated data will randomly generate 9-digit numbers to blind and protect Social Security numbers.
Even with a deidentified and HIPAA-compliant dataset, patients with a rare disease who have received a particular therapy are more easily reidentifiable simply due to the few number of patients with those same characteristics. With synthetic data, the same medical history data can be replicated but with randomly generated other data fields allowing for the same analysis but with reduced risk of patient reidentification.
While rare and ultra-rare diseases are a perfect example of this use case of synthetic data, the downstream effects are broader and more impactful. “If we can reduce risk without compromising the outcome by using synthetic data, it ultimately leads to increased data sharing — both internally as well as with external data partners,” says Davis. The value to the pharmaceutical industry as a whole, he notes, is efficiency. “If data sharing becomes more commonplace it becomes the fuel for true innovation.”
Use case #2: training AI models
Within the context of innovation, Davis says, is the most rapidly evolving technology of all, artificial intelligence. “If we can cheaply and reliably generate synthetic data, this data can most easily be applied to the training of AI models, which creates not only efficiencies but potentially increased accuracy of those models as well,” he says.
Because synthetic data has randomly generated data points but maintains the structural and mathematical integrity of the original dataset, it can actually remove potential biases when training an AI model. Furthermore, AI models can be “trained harder” with more diverse datasets rife with outliers. Ultimately, the use of synthetic data within AI model training will lead to increased accuracy as well as speed and efficiency of the training itself.
Use case #3: filling gaps in RWD
When choosing the proper datasets to analyze for a specific business question, there are almost always pros and cons that must be weighed. One dataset may provide the longitudinality required, another may possess the depth of acute care data necessary, but yet another dataset may be needed to address pharmacy claims data. To procure three separate databases and link them together takes significant time and investment, which are not always readily available.
By introducing synthetic data, existing real-world data can be augmented theoretically quicker and cheaper while still maintaining the statistical properties of true RWD. “In these instances,” Davis says, “we can potentially throw gasoline on the fire of RWD analyses and learn much more about our markets and patient populations, including deep root-cause analyses which can, in theory, revolutionize our approach to medicine.”
Sponsors may want to learn how a disease progresses within a specific patient population, how many times patients see a physician, exhibit a certain behavior, or what percentage develop a particular morbidity. For post-market studies, sponsors may seek to gather additional safety data on the marketed product. They want to understand how the product is being utilized in the real world but may lack a robust enough dataset to enable the statistical analyses required.
In terms of HEOR, sponsors want to determine the potential economic impact. In a non-interventional study, for example, how many hospitals were included, what did it cost the system, what drugs were used?
“In all of these cases,” Davis says, “we can significantly increase the efficiency of data procurement and analytics.” When it comes to statistical significance, it’s more about the trends than the data points, he adds.
“We are building additional tools for making this data much more accessible than it has been in the past. We’re at our infancy in this space, which affords us some flexibility and advantages.”
To see how Citeline is harnessing the power of AI for clinical planning and , visit Citeline.com.
1Arora A, Arora A. Generative adversarial networks and synthetic patient data: current challenges and future perspectives. Future Healthc J. 2022 Jul;9(2):190-193. doi: 10.7861/fhj.2022-0013. PMID: 35928184; PMCID: PMC9345230. Available from: Generative adversarial networks and synthetic patient data: current challenges and future perspectives - PMC (nih.gov) [Accessed Aug. 21, 2024].