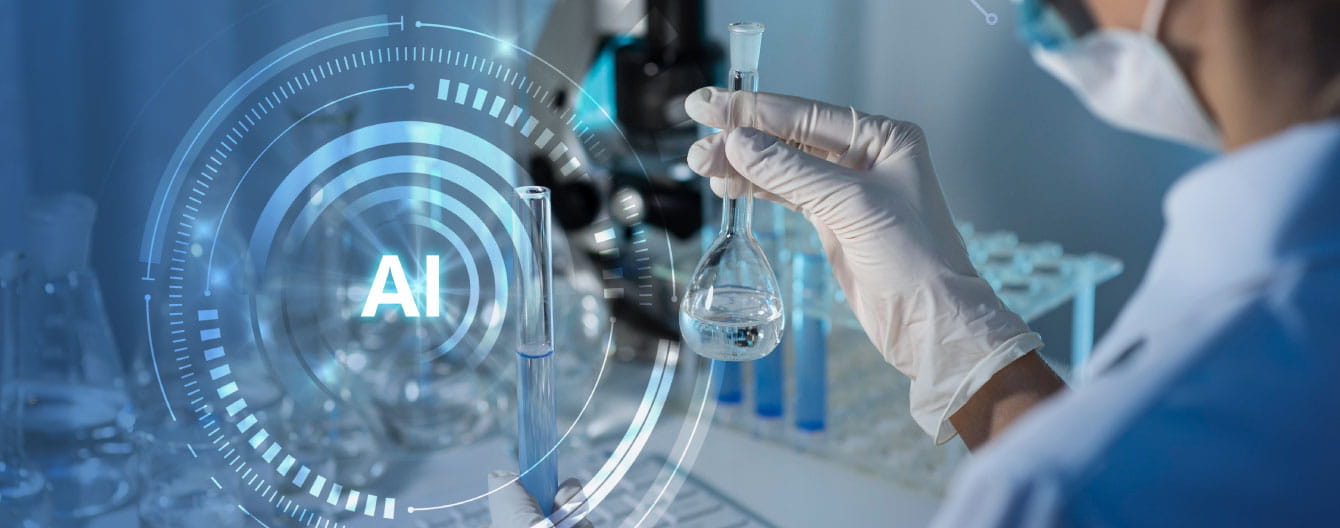
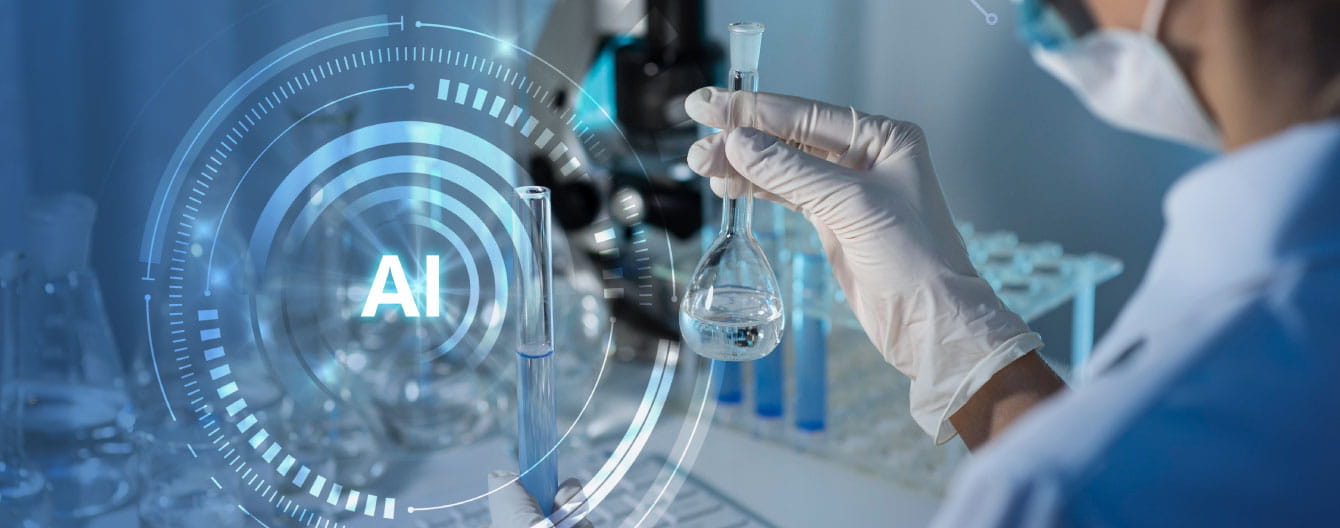
In clinical research, as with many industries, artificial intelligence (AI) is met with both excitement and trepidation. The impact of AI and machine learning (ML) is starting to be felt across the industry.
Artificial intelligence. There’s no arguing it’s a game changer. Some would say it not only rivals but surpasses the advent of human artificial insemination in the 1770s and artificial sweetener in 1876. In clinical research, AI is met with both excitement and trepidation.
As Claire Riches, VP of Clinical Solutions for Citeline, noted at the Citeline Elevate event earlier this year, the risks associated with the application of AI in clinical research are “the elephant in the room.” She posed the question: “What do we as an industry need to do to manage mitigate that risk?”
Leading up to that discussion, Suzanne Caruso, SVP New Product Strategy for Norstella, Citeline’s parent company, shared a brief overview of the state of AI and machine learning (ML) in the industry to date. In 2022, 175 trial submissions were supported by machine-learning models.
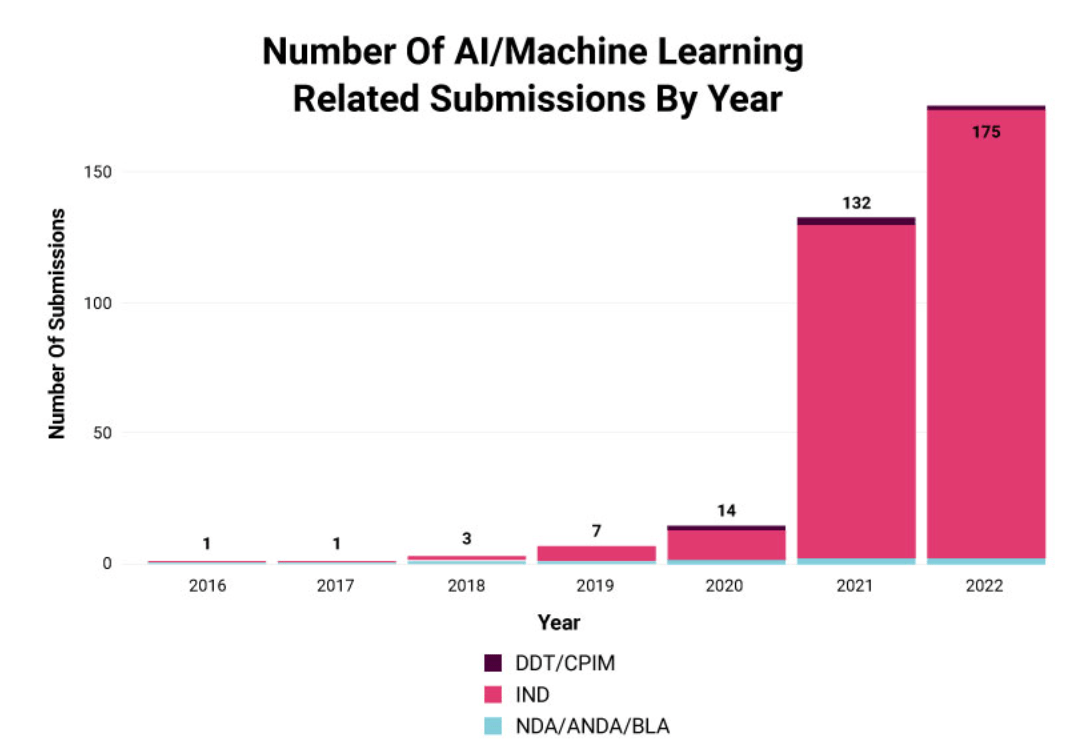
Source: FDA’s CDER Is Fostering Use Of Artificial Intelligence In Drug Development – Pink Sheet, Feb. 28, 2023
Caruso said the impact of AI and machine learning (ML) is starting to be felt across the industry. Citing eClinical Solutions’ 2024 Industry Outlook, looking at organizations integrating AI/ML in clinical data workflows, she noted:
- 80% are in the exploratory stage or planning to implement AI/ML
- 13% have no current plans to implement AI/ML
- 7% have integrated AI/ML across one or multiple applications
In a separate Citeline event, Caruso shared where the impact of AI is being seen in the drug pipeline:
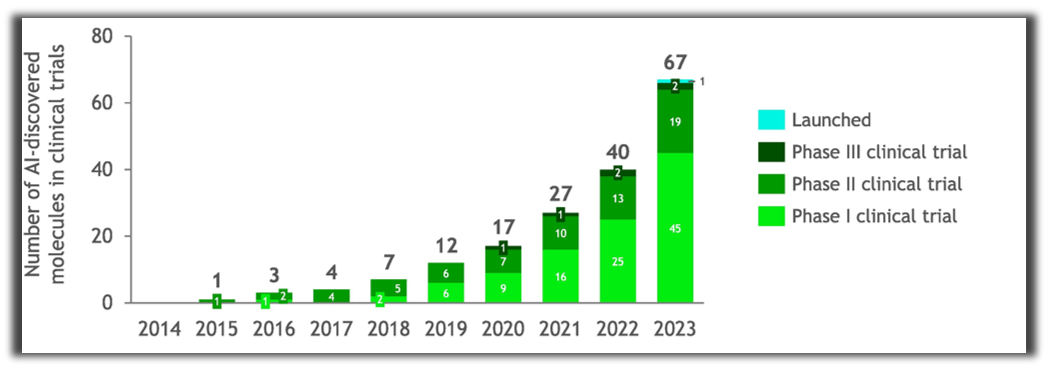
Since 2015, AI-native biotechs and their partners in the pharmaceutical industry have entered 75 molecules into the clinic, of which 67 were in ongoing trials as of 2023.
The pAIn points
Riches asked Baba Shetty, CTO of Syneos Health, to elaborate on the pros and cons of AI in clinical trials. He acknowledged AI requires certain guardrails that must be maintained.
Bias is one concern that Shetty said the industry has to address. “AI is only as good as the inherent training data on which it has learned from,” he said. “And if there is a bias inherent in the training data, then the AI system will in fact be biased.” It’s the garbage in/garbage (GIGO) concept regarding data science, the theory that in any system, the quality of output is determined by the quality of the input.
Shetty said another AI concern is the fundamental inconsistency of large language model-based systems, which he says is just a subset of the general topic of inconsistencies. “And we are not used to having systems in business, especially in our business, that deliver inconsistent results. We expect an answer and we expect that answer to be the same if I ask it today or next Tuesday.”
Safety is yet another issue, Shetty said. “So you have to monitor drift in these models,” he advised. “We just have to be constantly vigilant. We have to embrace the kind of state-of-the-art tools to ensure that we're getting the outputs that we need. And for us, the very simple rubric for right now is human always in the loop.”
Given these concerns, Riches asked Shetty: “Is there genuine movement and genuine trust to adopt AI models?”
“I think people are motivated, but they're wary,” Shetty said, likening it to walking a tightrope. “And I think they're obviously a highly regulated industry with a lot of implications to patient safety.”
Is AI worth the risk? Shetty is a believer. “The utility is just so, so incredible that it's well worth solving these problems,” he said. He sees great potential for AI in terms of both strategic and operational decisions in the clinical space.
Riches agreed, saying: “I think everyone can agree it's going to be part of our future in the pharma industry.”
Abigail Dirks, Data Scientist, Tufts Center for the Study of Drug Development (CSDD), emphasized the need to avoid putting AI technology in a silo. She said patient and community input, coupled with technology and data, can enhance the drug development process. This is particularly true, she noted, in generative AI-enabled clinical trial planning, design, and execution.
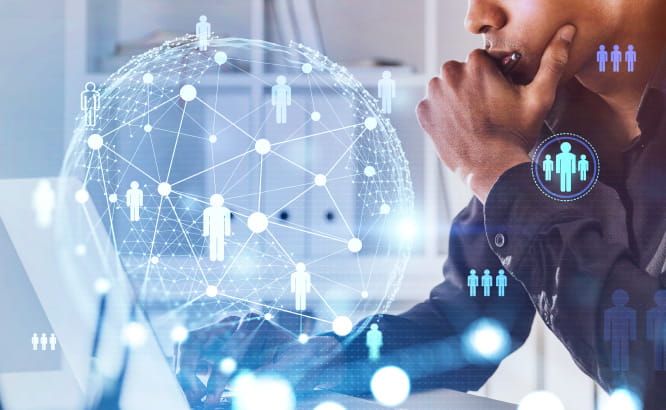
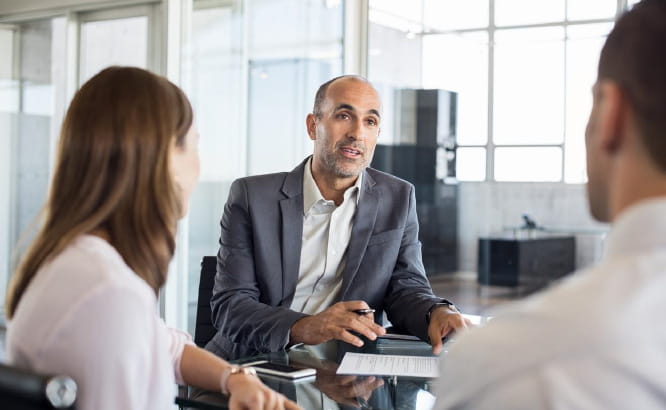
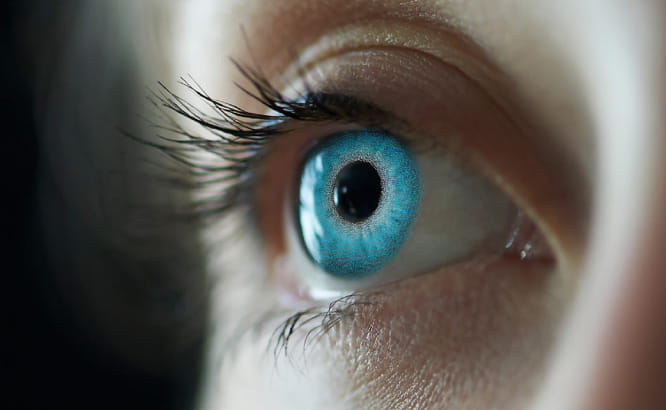